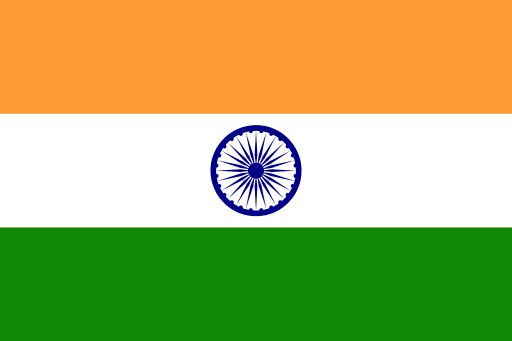
Über
I am Gopal Chitalia, a postgraduate from IIIT-Hyderabad(India). I have worked upwards of 1750+ hours on Upwork and on projects of tremendous diversity such as AI/ML, algorithmic trading, energy efficiency, software development, research papers, tutoring. I am also very active on codementor, helping multiple mentees Some of my clients include: Data Scientist - ClevAir, Norway Algorithmic Trading Developer - Tantra Labs, CA, USA Backtester & ML Expert - Hyperion Fintech, Zug, Switzerland ML Scientist/Energy Demand Expert - Growthworks.ai, CA, USA Research Assistant/Visiting ML Scholar - Smart Grid Research Unit, Chulalongkorn University, Bangkok One of my research problems on predicting short/long term energy prediction in office/residential buildings using machine learning is published at Applied Energy. The results improve the state of the art results by 20 to 40%. Applied Energy is one of the leading journals in the domain with an Impact factor of 10. I am also a reviewer at Applied Energy. I have also published a building level dataset paper in Nature Journal
I am Gopal Chitalia, a postgraduate from IIIT-Hyderabad(India). I have worked upwards of 1750+ hours on Upwork and on projects of tremendous diversity such as AI/ML, algorithmic trading, energy efficiency, software development, research papers, tutoring. I am also very active on codementor, helping multiple mentees Some of my clients include: Data Scientist - ClevAir, Norway Algorithmic Trading Developer - Tantra Labs, CA, USA Backtester & ML Expert - Hyperion Fintech, Zug, Switzerland ML Scientist/Energy Demand Expert - Growthworks.ai, CA, USA Research Assistant/Visiting ML Scholar - Smart Grid Research Unit, Chulalongkorn University, Bangkok One of my research problems on predicting short/long term energy prediction in office/residential buildings using machine learning is published at Applied Energy. The results improve the state of the art results by 20 to 40%. Applied Energy is one of the leading journals in the domain with an Impact factor of 10. I am also a reviewer at Applied Energy. I have also published a building level dataset paper in Nature Journal
Erfahrung
Developed Deep learning algorithms for predicting demand at CAISO region.
Worked on portfolio analysis to measure market impact of algorithm, comparing live algorithm to test.
Developed Machine learning models using RNN/LSTM for predicting day ahead building level energy consumption which helps in optimizing building operations and energy savings.
Developed a platform for load forecasting as a service.
Worked on reinforcement learning application for Demand Response applications in Smart Grid on Building & controlling HVAC systems for energy efficiency.
Developed a machine learning based methodology for building level-load foreacasting. This work has been accepted in Applied Energy Elsevier Journal
Built a mobile application for the web counterpart. Prototyped and developed the overall back-end of the application. The project was developed using NativeScript, TypeScript along with HTML/CSS/JS and RESTful API's.
Bildung
Projekte
This paper presents a robust short-term electrical load forecasting framework that can capture variations in building operation, regardless of building type and location. Nine different hybrids of recurrent neural networks and clustering are explored. The test cases involve five commercial buildings of five different building types, i.e., academic, research laboratory, office, school and grocery store, located at five different locations in Bangkok-Thailand, Hyderabad-India, Virginia-USA, New York-USA, and Massachusetts-USA. Load forecasting results indicate that the deep learning algorithms implemented in this paper deliver 20–45% improvement in load forecasting performance as compared to the current state-of-the-art results for both hour-ahead and 24-ahead load forecasting. With respect to sensitivity analysis, it is found that: (i) the use of hybrid deep learning algorithms can take as less as one month of data to deliver satisfactory hour-ahead load prediction, (ii) similar to the clustering technique, 15-min resolution data, if available, delivers 30% improvement in hour-ahead load forecasting, and (iii) the formulated methods are found to be robust against weather forecasting errors. Lastly, the forecasting results across all five buildings validate the robustness of the proposed deep learning framework for the short-term building-level electrical load forecasting tasks.
This paper describes the release of the detailed building operation data, including electricity consumption and indoor environmental measurements, of the seven-story 11,700-m2 office building located in Bangkok, Thailand. The electricity consumption data (kW) are that of individual air conditioning units, lighting, and plug loads in each of the 33 zones of the building. The indoor environmental sensor data comprise temperature (°C), relative humidity (%), and ambient light (lux) measurements of the same zones. The entire datasets are available at one-minute intervals for the period of 18 months from July 1, 2018, to December 31, 2019. Such datasets can be used to support a wide range of applications, such as zone-level, floor-level, and building-level load forecasting, indoor thermal model development, validation of building simulation models, development of demand response algorithms by load type, anomaly detection methods, and reinforcement learning algorithms for control of multiple AC units.